Previewing NeuralPLexer3: Towards fully AI-enabled Structure-Based Drug Discovery
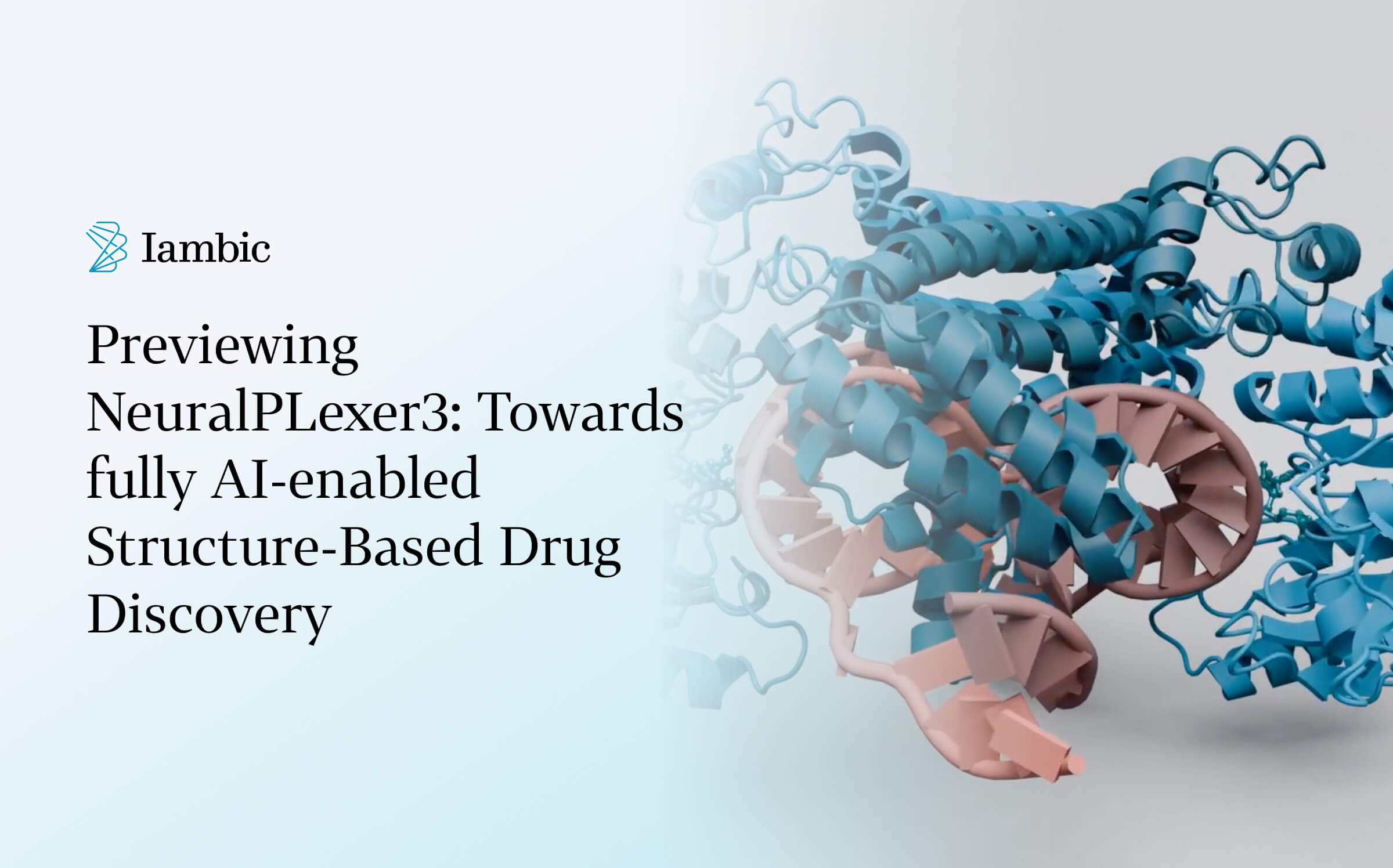
NeuralPLexer3 Beta sets new standards for accuracy and speed in protein-ligand structure prediction, transforming structure determination from a bottleneck into an interactive tool for drug discovery.
Understanding the three-dimensional structures of protein-drug complexes is fundamental to modern drug discovery and for understanding disease biology. Yet for decades, this critical insight has been bottlenecked by the limitations of experimental structure determination.
Traditional methods like X-ray crystallography and cryo-EM, while powerful, require weeks of specialized work and significant expense. AlphaFold kicked off a revolution in AI-driven structure prediction, but more progress is needed: Most drug development programs still rely on experimental structures for a small handful of key compounds, leaving countless therapeutic hypotheses unexplored.
Iambic has created NeuralPLexer to overcome this challenge, transforming protein-ligand structure determination from a bottleneck into an interactive discovery tool. When we first introduced NeuralPLexer, Iambic pioneered the use of AI for protein-ligand structure prediction. With NeuralPLexer2, we dramatically expanded these capabilities, enabling drug hunters to unlock challenging targets, identify cryptic binding sites, and elucidate complex mechanisms of action. Today, we're excited to preview Iambic’s next major advance: NeuralPLexer3 Beta.
Raising the Bar for Structure-Based Drug Discovery
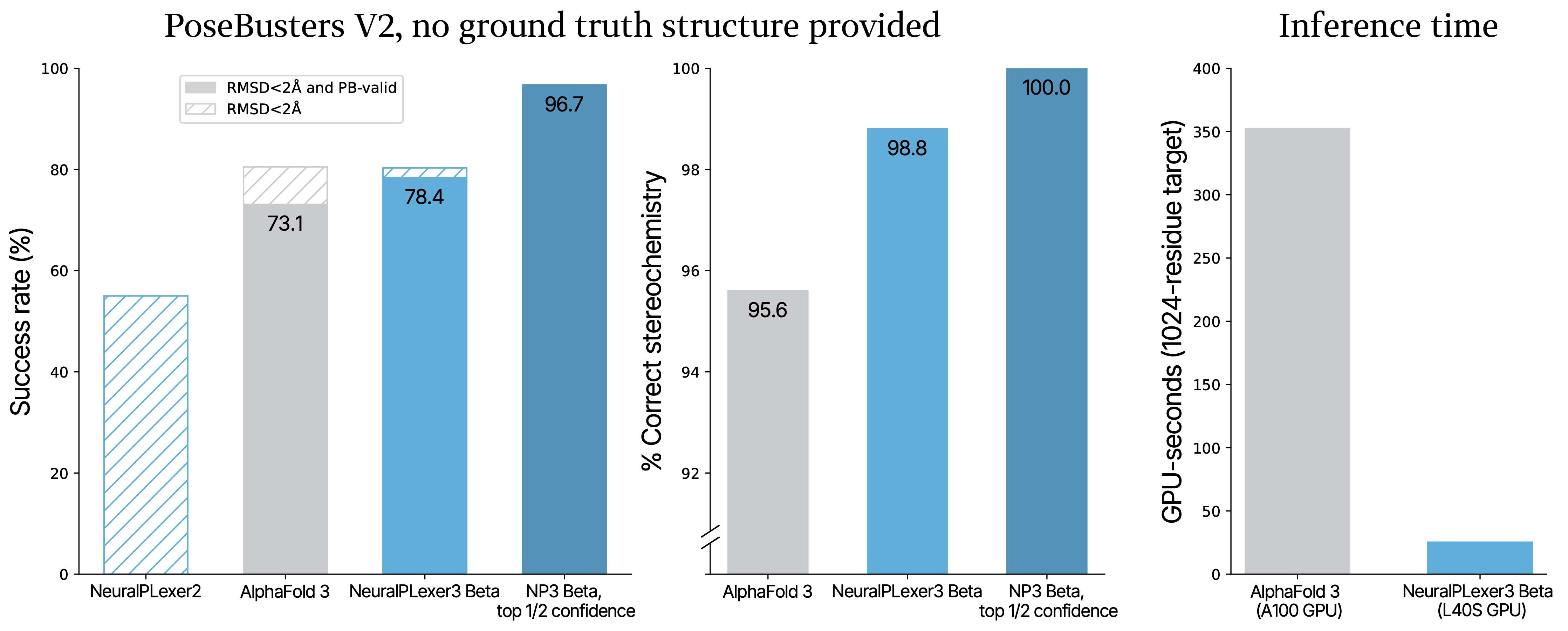
On the industry-standard PoseBusters benchmark, NeuralPLexer3 Beta achieves unprecedented accuracy in predicting protein-drug complexes. This benchmark evaluates two critical aspects that determine whether a model truly enables structure-based drug discovery: whether the predicted structures match experimental data to within the necessary accuracy (<2Å RMSD) and whether their molecular geometries are physically valid (PB-valid).
Starting with just a protein sequence and a ligand's chemical structure – without any prior structural information about the protein, binding site location, or ligand conformation – NeuralPLexer3 Beta achieves a 78% success rate on both metrics combined, surpassing AlphaFold 3's 73%. NeuralPLexer3 Beta is 97% accurate on the one-half of test structures for which it is most confident. The model also demonstrates 99% accuracy in predicting ligand stereochemistry, a crucial factor for drug design.
These predictions are delivered at unprecedented speed: 15x faster than AlphaFold3 for a typical system. While AlphaFold 3 requires specialized A100 GPUs and minutes of computation time, NeuralPLexer3 Beta delivers results in seconds on a single L40S data center GPU. This breakthrough enables scientists to rapidly test hypotheses and iterate on designs in real-time. The combination of speed and commodity hardware additionally makes it practical to screen millions of compounds on-demand, dramatically expanding the scope of structure-based drug discovery campaigns.
The Journey to NeuralPLexer3 Beta
.png)
This leap forward was propelled by systematic advances across our entire pipeline. The path to NeuralPLexer3 Beta involved innovations in data processing, model architecture, and computational efficiency.
We began by rebuilding our data pipeline from the ground up. Our new system processes the entire Protein Data Bank (PDB) with enhanced chemical annotations and metadata, enabling better handling of complex molecular interactions and providing a richer foundation for learning. The expanded dataset encompasses a broader range of biological structures and interaction types, contributing to the model's comprehensive capabilities. Indeed, NeuralPLexer3 Beta is trained on every atomic coordinate in the PDB (up to its training cutoff date).
The model architecture and generative modeling framework were redesigned to begin structure prediction from physically informed initial configurations rather than random noise, leading to more accurate predictions and faster convergence. We also made substantial improvements to our inference pipeline, incorporating physics-based guidance during the prediction process.
Finally, speed and efficiency were crucial focuses of development. Unlike traditional transformer architectures, the unique requirements of molecular structure prediction created special challenges for GPU optimization. One highlight is our novel FlashTriangularAttention mechanism, which dramatically reduces memory usage while accelerating model computation. With this optimization, NeuralPLexer3 Beta handles larger structures and delivers results faster than ever before, with peak memory usage reduced by 5x and model inference time improved by 50% in a typical case.
Comprehensive Coverage of Biological Structures
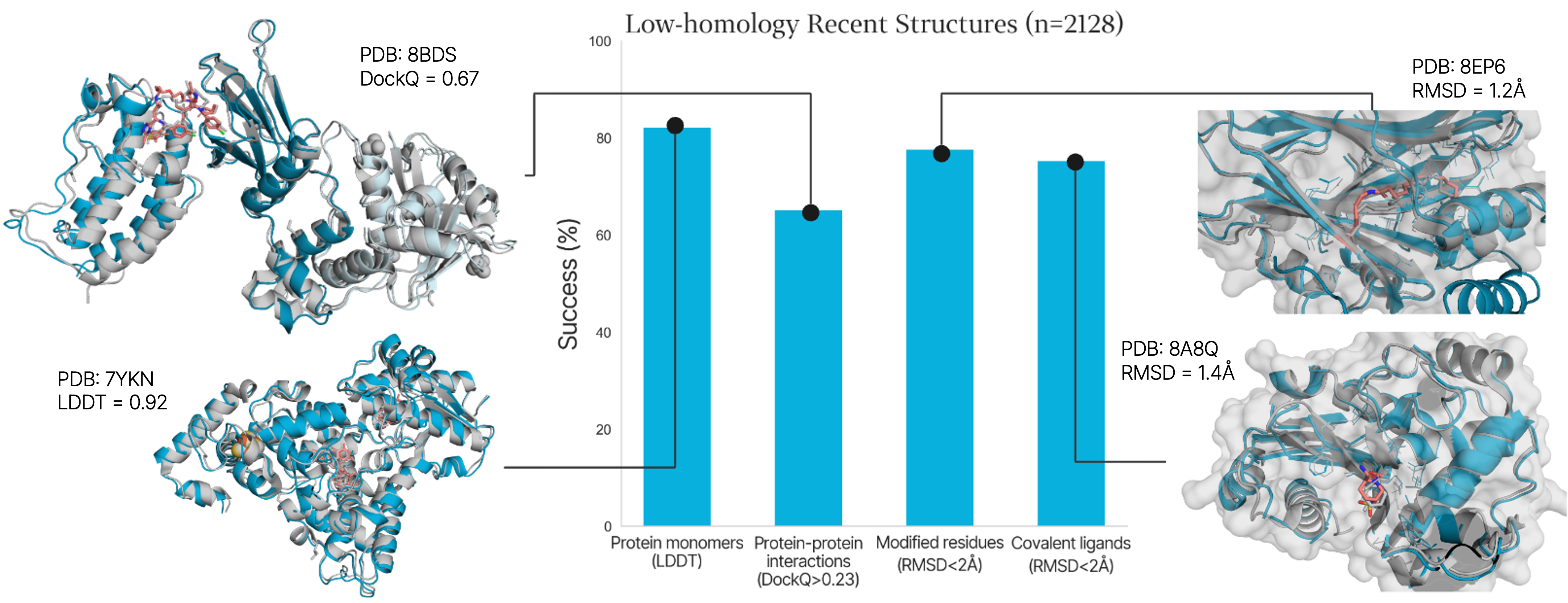
While accurate protein-drug structure prediction is our north star, NeuralPLexer3 Beta excels across the full spectrum of biological structures. This comprehensive accuracy isn't just a nice-to-have feature – it's fundamental to how the model works. To correctly predict how a drug binds, we need to understand both the local pocket structure and how binding might influence the global protein conformation. This creates a complex interplay where accuracy in one area reinforces accuracy in others.
NeuralPLexer3 Beta shows excellent performance across protein monomers, the bread-and-butter of structure prediction. It also achieves excellent accuracy in protein-protein interactions (PPIs), which are critical for many modern therapeutic strategies including PPI modulators, biologics, PROTACs, and molecular glues.
Better still, NeuralPLexer3 Beta demonstrates unprecedented accuracy for structures involving chemical modifications of proteins, whether through post-translational modifications or covalent ligands. This capability is particularly relevant for the growing field of covalent drug discovery, where accurate structural prediction can help design highly selective and effective therapeutic compounds.
Looking Ahead
NeuralPLexer3 Beta represents a significant step toward our vision of fully AI-enabled structure-based drug design. By providing instant, accurate structural insights across the full range of protein classes and drug molecules, we're fundamentally changing what it means to be structurally enabled in drug discovery. Our scientists can now explore therapeutic hypotheses at unprecedented speed and scale, transforming structure determination from a bottleneck into an engine of discovery.